What is Data Science?
- Data science is not a field of is own but a unification of statistics, analytical models and programming.
- A data scientist makes sense of data in order to provide added value for a given use case.
- It is common for data scientists to work closely with the business people. The role of the data scientist is to understand the need and transpose it to a data science solution.
- Data science is not an ordinary programming field (e.g. Developing a mobile application) but looks forward more towards research & development or trial and error.
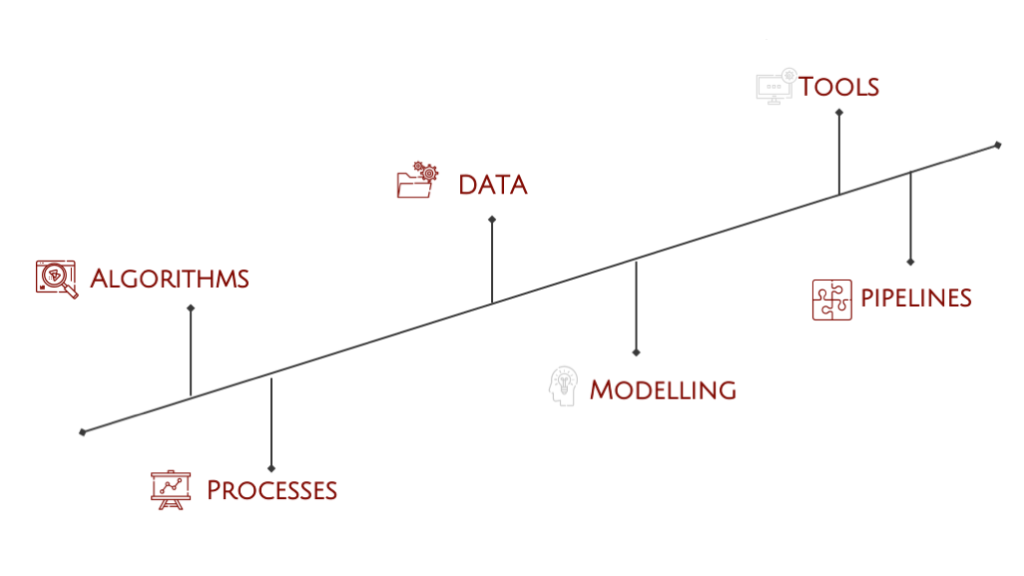
Data
- A Data scientist usually deals with two main categories of data which are:
- Structured (e.g. tables, jsons, csvs etc.)
- Unstructured (e.g. free text, images, videos etc.)
- Another important thing prior to applying any algorithm is if data is labeled. Labelling refers to assigning a semantic value to a given data instance (e.g. an Image of a cat, a scientific text etc)
- For most algorithms data need to be broadly organized in data points with a label or not. These need to be homogenous in terms of having the same “features” for structured data or being of a certain kind for unstructured (e.g. transactional data for structured and only images not mixed with text data points for unstructured).
Algorithms
- The algorithms for data science fall into two main categories: supervised and unsupervised learning. Supervised learning means trying to “learn” a given human annotated label and unsupervised learning also “learns” but not towards a given “grund truth” aka the label.
- To oversimplify all algorithms are advanced statistical models aka “machine learning” .
- They further diversify into regression and classification for supervised learning and clustering/anomaly detection for unsupervised.
- Some notable algorithms are SVM/Decision Tree for “classical” machine learning as well as Neural Networks for novel deep learning which have a multitude of variations being a field of its own.
Processes
- This section refers to the methods which are applied after data is established for a model development. Real life data is usually messy and needs to be further processed in order to be usable.
- Such processes are imputing which is dealing with missing values, smoothing which is most of the time transformation of the data when it is too variant, cleaning which deals with tweaking different features (e.g. deleting un meaningful columns in tabular data, fixing upper/lower case in text, splitting a column in two etc).
- Another important process is feature generation (e.g. aggregating data, TFIDF, PCA, ONE-HOT-ENCODING etc).
Modelling
- Modelling is the core activity of the data scientist and the “end product”. In order to model something multiple algorithms are put on “trial” for “learning”.
- Usually this is not straight forward, and multiple iterations are necessary as well as going back to previous steps.
- In a nutshell, a model is a “black box” that is able to generalize a given topic and provide high probable and qualitative result (e.g. which images are likely cats, is a customer going to churn on the business, is this person not like the others etc).
Pipelines
- Apart from different processed that may arise with a problem at hand, the data science process has a string of pipelines that can be generalized.
- ETL stands for Extract, Transform, Load and it is the data acquisition step that bundles all data from eventual multiple sources.
- EDA stands for Exploratory Data Analysis and is getting a visual introspection into the data (e.g. graphics, statistics, dependencies etc).
- DQ stands for Data Quality and the scope is to fix/drop/evaluate the quality of the data which the data scientist got.
- Serving and Deployment are usually done by MLOps team, however most of the time in tight collaboration with the datascientist.
Tools
- In term of tools there is a big open-source market of it, however there are some recommended state-of-the-art ones and which have the biggest communities.
- The backbone of data science is Python programming language.
- For algorithms there is tensorflow for deep learning and sklearn for multiple other.
- Pandas and SQL are musts for ETL.
- Plotly/Matplotlib are very nice graphical libraries for EDA.
- Spark/Hadoop/Kafka are solutions for handling big chunks of data and streaming as well as ETL.
- Airflow is best in class solution for orchestrating/deploying/serving/automating production ready models.