Context, Trends, Movements
The Analytics space is an ever-changing subject which requires a fast pace and a mindset focused on building pilots, testing new features and analysing compatibility with present infrastructure for any organisation that desires a data-driven decision making process. The R&D movement which more and more organisations are implementing is usually prone to failure due to lack of patience and desire to build ready for production units in a strict time frame. It is desired by executives and embraced by developers, although most results lack real world applicability or simply do not improve/ add value to current processes or products. While this can be traced by following the decision making process, sometimes this happens due to a cumulation of factors such as: infrastructure incompatibility, lack of iterative developments, final result focus, no real world testing and so on.
“What you will be working on 10 years from now has not been invented yet.”
Small platforms are making their way into management mainstream following the adoption of a more agile way of work. The change has different implications that surpass the infrastructure or tools used – this affects primarily the development cycles and product testing. Mammoth analytics infrastructures are slow, heavy and usually require additional know-how to operate and configure them, thus raising valid concerns for the management in terms of profitability and organisational adoption. Building, testing and deploying new Machine Learning products should not be seen as a milestone or a great accomplishment by the executive level rather a new tool/ asset for the organisation in order to accomplish desired KPIs. This change in mentality has a lot of bridges to cross in order to be successfully implemented. Bigger is not necessarily better in terms of testing and developing new Analytics products, but also we acknowledge the fact that too small of a platform can greatly impact the pool of models used and usually comes with intensive memory optimisation.
What’s the concept?
The go-to tools and solutions for Analytics developers are usually the Open Source ones – some with overwhelming adoption by the community (e.g. Jupyter Notebooks). Building and testing Machine Learning solutions does not require heavy solutions, rather an IDE and a programming language that supports/ has implemented some ML libraries. Organisations that recently invested in an analytics team have a high chance of using the same solutions that a student does for his homework: simple IDEs, maybe a model repository (usually MLflow) – or just pickle (used to serialise objects, e.g. save models to a file) and a Database connection which in some instances is successfully represented by an exported CSV file.
Usually the management has a certain amount of restraint in updating or building an analytics infrastructure without any prior results, profits or maybe valuable insights delivered. Which makes sense from our point of view. You do not need state of the art capabilities to retrieve some insights or maybe provide a different view for the business in order to optimise or create new processes. We consider that the problem arises when scaling the solutions as there is quite a difference between 1 model and 100 models developed. Of course, you can probably do it manually too, but the costs are high and the human resource scarce, as the developers are not keen on manual runs or file-based model management.
What does it take?
Building an infrastructure from scratch should not be a tedious task, especially considering the fact that the problem arises on integration with inplace systems. Best on-demand, auto-ML scalable infrastructure with zero to minimal integration will be more of a burden than an asset. No matter the budget or the capabilities, if you need to manually import and export a CSV file in order to process it and then upload your results to a Sharepoint there is no point in discussing scalability or real world impact other than some isolated use cases.
An in-house Analytics Platform should focus on a few standard aspects and several others that differ from one organisation to another. You need a development place, a repository for your code and one for your ML developments, an orchestrator/ scheduler and a tool for EDA (Exploratory Data Analysis). All combined with a full integration between the platform and desired input/output systems. From experience, I would recommend on backlogging for future developments an explanatory module for your projects and an auto-ML framework, which can easily be integrated by the team through python packages (ex: pycaret). Considering the fact that most solutions (if not all) can be found as open source containers, there is an extensive flexibility for the team to build and test suitable solutions for their organisation or even customise them with in-house plugins/ extensions.
One could argue that the adoption of open source systems in a closed proprietary environment can have various consequences, especially in terms of compatibility and lack of 3rd party support, but this is easily avoided as the platform does not need extensive integration, rather than open communication. Usually, the exchange will be done through APIs and will not in affect in any way how in-place systems behave. This is a strong asset to have. A flexible jack of trades that can enhance and produce valuable insights for the organisation in a rather short time frame.
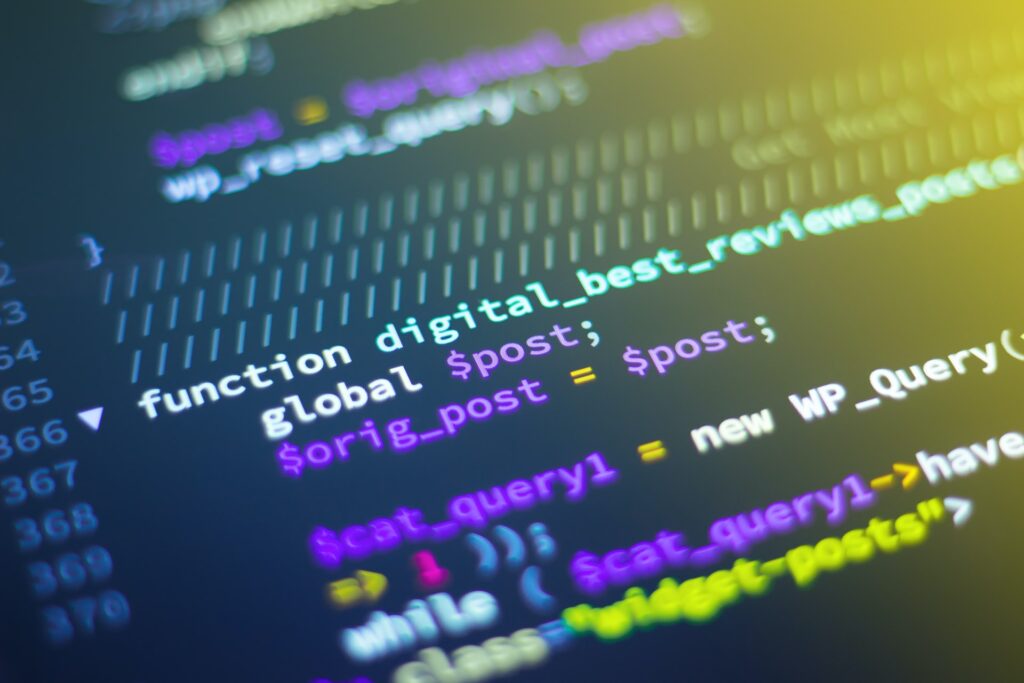
First steps in Open Source Technologies
World of open source technologies is vast and can be overwhelming when browsing without guidance. I recommend searching for the most used solutions, with an extensive community and recurrent updates. Also, browsing through the Apache community top projects can reveal some interesting tools (see Superset, Airflow – as a fun fact, both came from Airbnb™ and also from the same person: Maxime Beauchemin). Whatever tools and solutions you choose for your platform, keep in mind that the goal is to provide new and exciting insights for the organisation and also new capabilities and know-how for your team, department and business.
A step in the future
A quote that has stood with me since University sounds a bit like this: “What you will be working on 10 years from now has not been invented yet.”. Probably the sentence is not 100% bullet proof, but it strongly reflects the Data scene that we are experiencing first hand. The Data Management ecosystem will slowly but surely change its organisational aspects by absorbing various specific roles into a much broader general role as a “data person”. Technical analysts, developers, data engineers and so on, all these roles that now serve a specific purpose will most likely morph into a generic jack of trades one. Data Science & Data Analytics will be seen as indispensable as SQL & Data Warehouses. Clusters, segments, ad-hoc data driven analytics, forecasts, all these methods will become the norm, just as querying the Database is today. We will look back and ask ourselves why we left important strategic decisions to be made by relying on business expert decisions and not by automated data-driven processes. The organisations will need to be swift and adapt to the new landscape or suffer the same fate as heavy silos are having today: adoption of already burned out technologies as “state of the art”, mainly heavy & slow Data Lakes with 2012 technology stack.